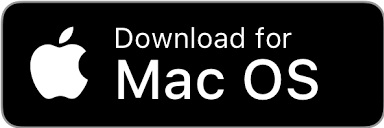
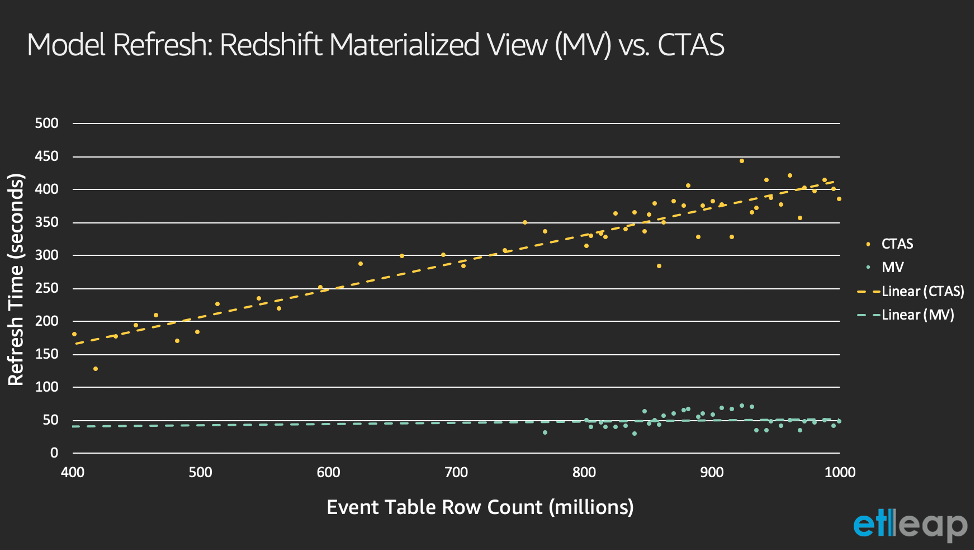
Materialization just implies that the transformation is done proactively. Think back to how regular views work: results are constantly changing as the underlying data changes.
#Redshift materialized views update
In principle: Materialized views should update automatically. A "view" implies an anchored perspective on changing inputs. A few have implemented automatic updates, albeit with long lists of limitations.

Some databases have materialized views that must be manually refreshed. In practice: It would seem there is no consensus. Should materialized views update automatically? The process of updating a materialized view in response to these changes is called view maintenance. Once a view is materialized, it is only accurate until the underlying base relations are modified.
#Redshift materialized views plus
If a regular view is a saved query, a materialized view is a saved query plus its results stored as a table. A materialized view is like a cache - a copy of the data that can be accessed quickly. Consequently, database accesses to the materialized view can be much faster than recomputing the view. Index structures can be built on the materialized view. What is a materialized view?Ī materialized view takes the regular view described above and materializes it by proactively computing the results and storing them in a "virtual" table.Ī view can be "materialized" by storing the tuples of the view in the database. In almost all modern databases, you can also "stack" views: You can create a view that references another view. You can then run queries against these derived tables from a BI tool, rather than from the atomic tables themselves.SELECT user_id FROM user_purchase_summary WHERE lifetime_value > 500 Įvery time the database gets a query referencing a view, it needs to first compute the results of the view, and then compute the rest of the query using those results. Each time new data is loaded into Redshift, the SQL queries are run, and the set of derived tables gets updated with the latest data. You can use SQL Runner to schedule SQL queries to run as part of the Snowplow pipeline. Rather than create a view, I’d run the exact same SQL and create a table instead (it would in effect be a materialized view). constraining a SELECT FROM query on a view with a WHERE clause is slower than if the view itself was defined with that same WHERE clause the Redshift query planner doesn’t optimize through views - so e.g.views are hardcoded to the table, not the table name, and difficult to update (if we need to recreate a table in atomic, all views that use that table will break).views are not materialized, so there is no inherent performance benefit.We recommend against using views in Redshift.
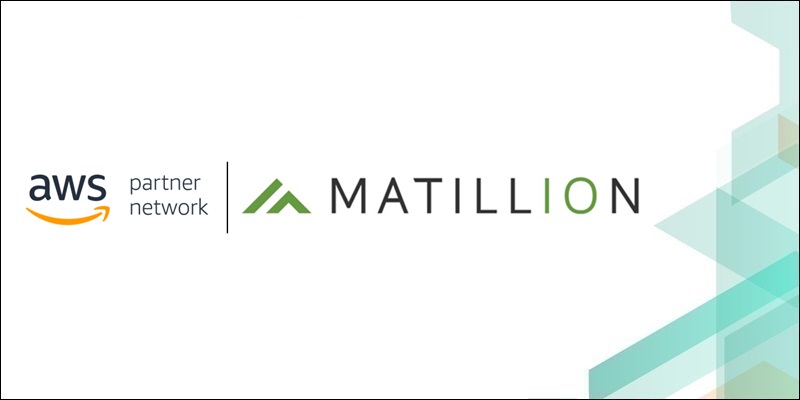
The answer might benefit other users as well, so we’re cross-posting it here. Additionally, we would like to know what your thoughts are on creating materialized views? I was wondering if you could offer some advice on the general performance of views in Redshift? We are finding them slow, and would like some pointers on what to do and what not to do. We received the following question from one of our customers:
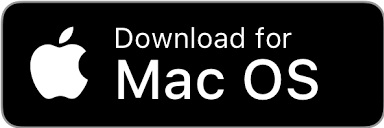